Understanding Mode Data Analytics: A Comprehensive Overview
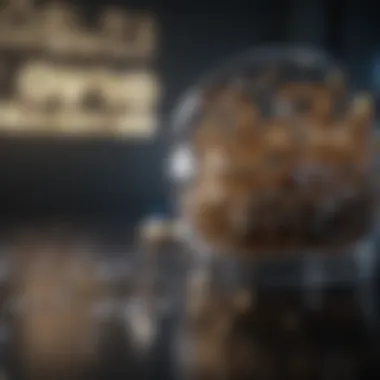
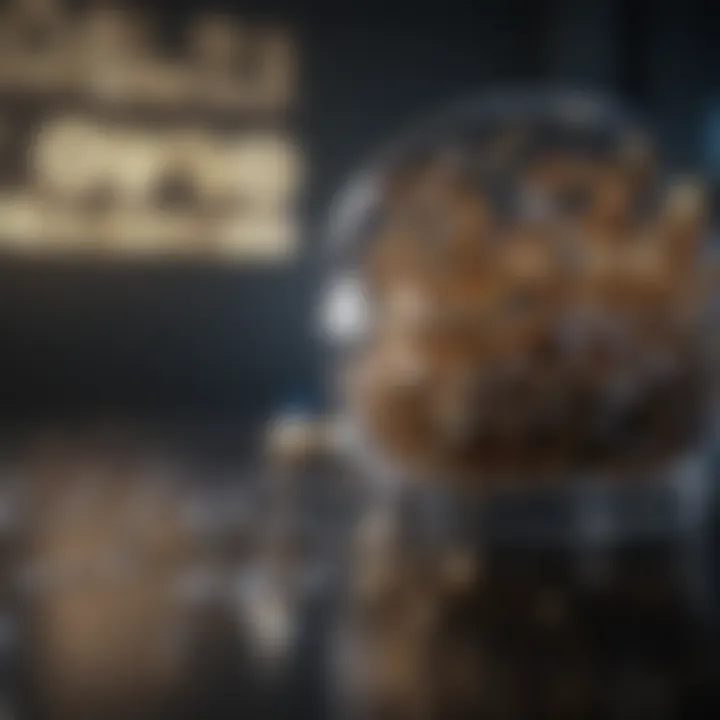
Intro
In the rapidly evolving world of data science, understanding the key components of data analytics has become crucial, especially for individual investors and financial professionals. Mode data analytics is a significant element among these components, representing a statistical measure that offers unique insights into data sets. By concentrating on the mode, or the value that appears most frequently within a data range, we can unveil patterns and trends that may not be immediately evident when examining means or medians.
As we navigate through this exploration, it will be vital to grasp the terminology related to investment, which lays the groundwork for deeper understanding. This knowledge not only enhances the decision-making process for investors but also augments overall financial literacy. By weaving in both theoretical foundations and practical applications, this discourse aims at shining a light on how mode data analytics operates in various contexts, particularly in finance.
Preface to Mode Data Analytics
In today’s data-driven world, understanding how to extract meaningful insights from data sets is vital. Mode data analytics plays a crucial role in this process, providing valuable information that can enhance decision-making, particularly in financial contexts. By centering on the statistical measure of central tendency known as the mode, this approach helps analysts and investors unearth patterns that might otherwise go unnoticed.
Definition and Importance
Mode data analytics, in simple terms, refers to the practice of determining the mode within a dataset. The mode is the value that appears most frequently, making it an essential measure for understanding trends and behaviors. Unlike other statistical measures such as mean or median, which can sometimes skew data if outliers are present, the mode remains sturdy—it reflects the most common observation.
The importance of mode data analytics is manifold. First, it provides a clear picture of what is typical in a dataset, which is particularly useful in sectors such as finance where understanding customer behavior can lead to better service offerings and targeted marketing. Investors can analyze purchase habits or transaction frequencies to gauge where opportunities might lie—after all, knowing what draws customers most can be half the battle in achieving sales targets. Furthermore, focusing on the mode allows analysts to streamline their approach, making complex data more digestible and easier to analyze without losing the essence of the underlying information.
The mode may not always be the star of the statistical show, but it often reveals what’s hiding right under the surface.
Historical Context
Mode data analytics isn’t a new concept, and its roots can be traced back to the early days of statistical discovery. Figures like Karl Pearson in the 19th century contributed to the central tendency studies, but the common usage of mode truly burgeoned in the mid-20th century when data became more accessible through technology. The advent of computers accelerated the ability to process and analyze large data sets, making it easier for businesses to implement mode analysis as part of their strategic frameworks.
Throughout the years, as industries began to embrace data science, the application of mode analytics grew exponentially. From retail trends to economic forecasting, practitioners started to realize the significance of understanding the most frequent occurrences in their fields. As data became more sophisticated, so did the tools and methods for analyzing it, pushing mode analytics to the forefront in various disciplines, especially in finance.
In summary, knowing the historical trajectory and importance of mode data analytics sets the path for deeper exploration. The subsequent sections will delve into more advanced frameworks, calculations, applications, and challenges, reinforcing how this statistical measure holds the potential for insightful revelations across sectors.
Theoretical Framework of Mode Analytics
The theoretical framework of mode analytics provides a fundamental understanding of how this statistical measure operates and why it matters in the realm of data analysis. It serves as a backbone for grasping the nuances of how the mode functions in relation to other measures of central tendency such as mean and median. It’s essential for data enthusiasts, especially financial professionals, to appreciate the context in which the mode is used as it significantly bolsters the decision-making process.
When examining data sets, the mode can often illuminate patterns that may otherwise be overshadowed by more prevalent averages. Unlike the average or mean, the mode focuses on the most frequently occurring values, thereby providing insights into the preferences or commonalities within data sets. This orientation can lead to effective market assessments and predictions, especially in fields where understanding consumer behavior is paramount.
Statistical Measures Overview
Mode data analytics thrives upon a set of statistical measures that help to dissect and interpret data. These measures include mean, median, and of course, mode itself. While each plays its own role within the analytical spectrum, they also interlink to form a cohesive understanding of data trends. Here are key aspects of these measures:
- Mean: Often seen as the average, the mean is calculated by adding all values and dividing by the number of values. It’s widely used but can be skewed by outliers.
- Median: The median is the middle value when data is organized in order. It provides a better sense of central tendency in skewed distributions.
- Mode: The mode highlights the most frequently occurring number in a dataset. It can be particularly useful in categorical data analysis where understanding group characteristics is beneficial.
Central Tendency Explained
The concept of central tendency encompasses a trio of statistical measures that serve to summarize data in a meaningful way. It gives the reader a 'central' or 'typical' value around which other data points cluster. Each measure has its own strengths and weaknesses, influencing which is best to use in particular situations.
Mean
The mean is a central figure summarizing the data points. It’s often favored in many analyses for its simplicity. However, the key characteristic of the mean is also its vulnerability to large outliers which can distort its representation of the data set as a whole. For instance, when assessing the income levels in a population, a few exceptionally high incomes can disproportionately raise the mean, leading to misconceptions about overall economic health.
Unique Feature: The mean is unique in that it incorporates every data point in its calculation, allowing it to be sensitive to changes in data, but this can be viewed as a double-edged sword.
Advantages:
- Easily calculable
- Works well in normally distributed datasets
Disadvantages:
- Sensitive to outliers which can skew results significantly
Median
The median’s strength lies in its resilience against outliers. By focusing on the middle value, it provides a balance that can better reflect a dataset’s characteristics when the distribution is skewed. For example, in the context of real estate prices within a given area, the median price can often give a more accurate picture than the mean, which might be influenced by a few exorbitantly high listings.
Unique Feature: The median’s ability to dismiss outlier effects makes it a reliable choice, especially in datasets that are not symmetrically distributed.
Advantages:
- More representative in skewed data than the mean
Disadvantages:
- Does not take into account the magnitude of values, just the order
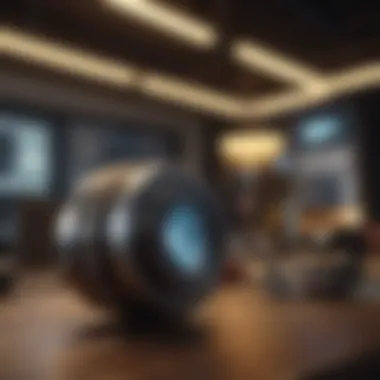
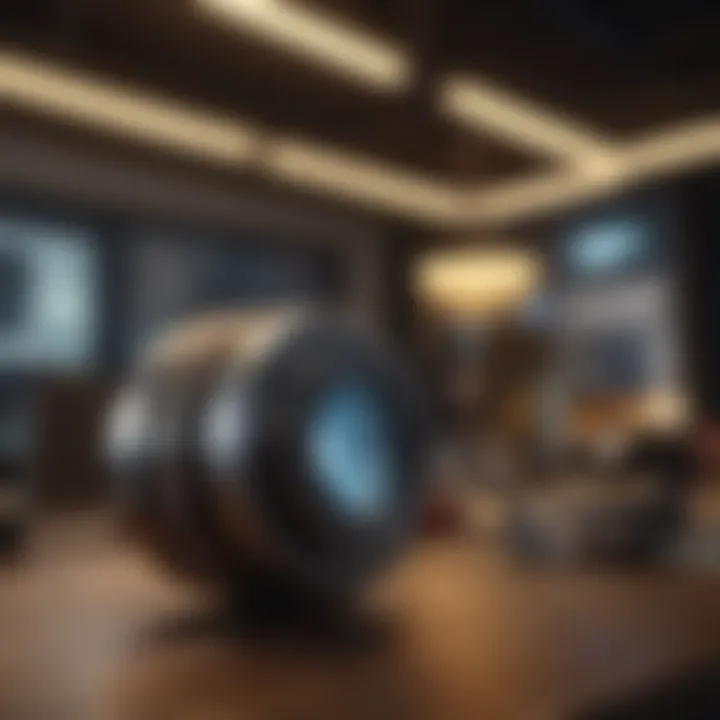
Mode
The mode serves a specialized role in the realm of statistics. It identifies the value that appears most frequently in a dataset, making it incredibly useful for categorical data where frequency counts are essential. For instance, a market researcher may find that a specific model of smartphone is the most purchased, which can direct marketing strategies effectively.
Unique Feature: The mode can also be used on non-numerical data. This versatility makes it handy in various fields.
Advantages:
- Useful for identifying trends and preferences in datasets
- Can be applied to non-numerical data such as categories or labels
Disadvantages:
- A dataset may have more than one mode or none at all, which can complicate analyses
Ultimately, the theoretical framework surrounding mode analytics aids investors and analysts in making sense of complex data. Recognizing the strengths and shortcomings of these statistical measures enables more informed choices, equipping decision-makers to sift through data effectively.
Understanding Mode in Data Sets
In the realm of data analytics, understanding the mode is pivotal for extracting meaningful insights from datasets. The mode, which is defined as the value that appears most frequently within a dataset, provides a unique lens through which analysts can gauge patterns and trends. It serves as a bridge between pure numerical representation and the narrative that data can tell, making it especially relevant for financial enthusiasts and individual investors.
Identifying the mode not only allows analysts to summarize data effectively but also sheds light on consumer behavior, product preferences, and market trends. Consider, for example, a retailer observing sales data. If the mode of a specific product sold indicates it is the most favored among shoppers, strategies revolving around that product can be adjusted for maximized profitability. This understanding extends beyond mere numbers, allowing for enhanced decision-making and strategic planning.
Calculating the Mode
Sample Data Analysis
Sample data analysis acts as the foundation for calculating the mode. Here, analysts typically extract insights from a representative subset of data instead of analyzing entire datasets, which can be procces-intensive. The key characteristic of sample data analysis lies in its efficiency; it provides ample knowledge without requiring exhaustive computation. This method is particularly beneficial for preliminary investigations or exploratory analysis.
For instance, if a financial advisor wants to assess the mode of investment preferences among a group of clients, they may collect data from a small representative sample rather than polling every client. One distinct advantage of this approach is that it saves time and resources while still offering actionable insights. However, caution is required—if the sample isn’t truly representative, conclusions drawn about preferences or behaviors may be misleading.
Frequency Distribution
Frequency distribution plays a critical role in understanding how data points are spread across various categories, highlighting the count of occurrences for each value in a dataset. This is particularly useful when investigating the mode, as it directly illustrates the frequency of each value. The hallmark of frequency distribution is its visual representation, often displayed in histograms or charts, making complex data digestible.
This unique feature allows analysts to spot trends at a glance—something raw data often obscures. Frequency distribution is a favorable choice for data representation in this context, as it encapsulates both the mode and the overall distribution of data in an intuitive manner. But it is not without drawbacks. For instance, while it paints a clear picture of frequency, it can sometimes oversimplify data, masking subtler insights that might be hidden in the finer details.
Interpreting Mode Results
Interpreting the mode results is where the magic happens. It transforms mere numbers into a narrative. Understanding the implications of what the mode reveals can lead investors and analysts to make informed decisions based on empirical evidence rather than gut feelings. The mode can point out not only what is popular but also hint at underlying trends over time, crucial for forecasting.
Directly correlating the mode with other key metrics like median and mean can enrich the understanding of a dataset even further. While the mode shows what’s most frequent, the mean provides an average view, and the median splits the dataset into two halves. Together, these metrics weave a comprehensive story that can inform strategic financial decisions.
"The mode is a crucial signal. It suggests where to focus time and resources, enabling timely and effective responses in a dynamic marketplace."
In essence, grasping mode results transcends basic statistical analysis. It aligns with broader business goals and fosters a data-informed culture among stakeholders, enhancing not only decision-making but also strategic planning across the board.
Applications of Mode Data Analytics
Mode data analytics plays a pivotal role across various sectors, with distinct applications that aid in decision-making processes. Understanding how mode serves as a tool to derive insights can not only streamline operations but also enhance strategies tailored to meet specific business needs. Whether it’s tracking customer preferences or measuring operational efficiency, the importance of mode becomes palpable in practical settings.
Financial Sector Analysis
In the financial world, mode analytics finds its footing in several critical applications. A discerning investor or financial analyst often looks to mode not just as a statistical figure but as a means to identify patterns in data. For instance, when analyzing customer transaction data at a bank, understanding the mode can reveal the most common transaction amount. This information can inform marketing strategies and operational enhancements, allowing the institution to cater to the prevalent needs of its customers.
Here are some specific ways mode data analytics influences the financial sector:
- Risk Assessment: Analyzing historical data around loan applications can help organizations identify the mode of default amounts, guiding more informed risk management.
- Portfolio Management: Investors can leverage mode to identify the most frequent returns on specific assets, thus optimizing their investment strategies.
- Customer Segmentation: By identifying the mode of customer transactions, businesses can develop targeted offers that align with the majority's preferences.
Market Trend Evaluation
Meanwhile, in the realm of market trend analysis, mode serves as an invaluable asset for detecting shifts in consumer behavior. For market analysts, knowing the most common prices for certain products or commodities enables a sharper focus on pricing strategies. This can in turn affect supply chain decisions and promotional activities.
Consider some aspects of market trend evaluation driven by mode:
- Consumer Behavior Insights: Understanding the mode of purchasing patterns during specific sales periods gives companies the ability to predict future buying behaviors.
- Competitive Analysis: By examining the mode of price points offered by competitors, businesses can strategize their pricing and promotional efforts to exploit market gaps.
- Stock Market Analysis: Tracking the modal price of stocks over a set timeframe can help investors gauge market conditions and make predictions about future movements.
The savvy utilization of mode within data analytics transforms raw numbers into actionable insights that can significantly enhance financial decision-making.
Mode vs. Other Statistical Metrics
The mode, often positioned as the overlooked sibling in the family of statistical metrics, plays a pivotal role in data analysis. It can sometimes be lost in the shadows cast by mean and median, yet its merits are simple and profound. When diving into mode analytics, it's crucial to compare it with other central tendency measures to paint a clearer picture of its significance.
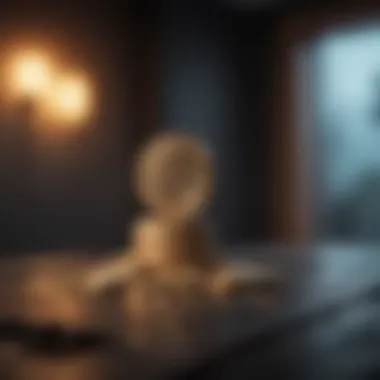
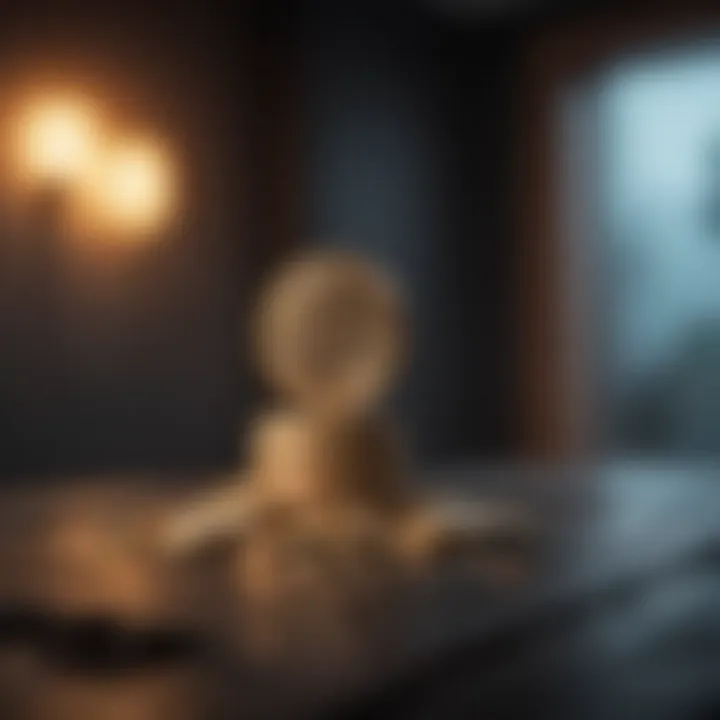
Comparison with Median and Mean
When considering how mode stacks up against the median and mean, one must look at their fundamental differences in calculation and applicability. Mean is what most think about when discussing averages. It’s determined by summing up all values and dividing them by the count of these values. This makes it sensitive to outliers, leading to skewed results in datasets with extreme values.
Median, on the other hand, is quite a different beast. It simply represents the middle value when the data is sorted. This means it's less affected by outliers and can often present a clearer picture of central tendency in certain distributions.
Mode enjoys a different kind of attention. It's the value that appears most frequently in a dataset. This can be particularly useful in categorical data analysis, where mean and median may not apply at all. For instance, when analyzing consumer preferences, identifying the most popular product or choice can illuminate trends that lots of investors may overlook.
To summarize:
- Mean: Sensitive to outliers, best for continuous data.
- Median: Resilient, good for skewed distributions.
- Mode: Essential for categorical data and identifying trends in frequency.
"Numbers may lie, but the mode tells the frequency of choice, showcasing what people really prefer."
Each measure has its strengths and weaknesses, which makes understanding their nuances critical for accurate data interpretation. Another point worth mentioning is that datasets may have more than one mode. In such cases, we refer to it as bimodal or multimodal, while mean or median only yield one value regardless of distribution.
Limitations of Using Mode
However, despite its unique benefits, mode comes with limitations. One primary concern is its lack of robustness. In datasets where values are evenly distributed, one might find it challenging to pinpoint a mode, or it may not represent the data trends effectively. Imagine a company selling numerous currencies; if each currency is bought equally, the mode might not offer valuable insights.
Another issue involves its applicability in numerical datasets. While it shines in categorical cases, relying solely on mode in continuous numerical data can sometimes mislead analysts. For instance, in a dataset documenting human heights, where values vary widely, mode may not convey the full story that mean or median undoubtedly would.
In summation, navigating the statistical landscape often requires selecting the right tool for the job. Recognizing when to employ mode versus mean or median can greatly influence data-driven decisions. While the mode provides a unique lens on frequency and trends, it's only one part of the equation that encompasses meaning in data analysis.
Tools and Technologies for Mode Analysis
The exploration of tools and technologies in mode data analytics is essential for harnessing the power of data interpretation. With the growing complexities in data sets, there’s a need for robust and intuitive software that can assist analysts and investors alike in their decision-making processes. Effectiveness in discovering the mode helps in identifying key trends and patterns critical to guiding future strategies.
Statistical Software Overview
Statistical software plays a pivotal role in mode analysis, enabling users to process large amounts of data swiftly and accurately. Two leading choices in this realm are R Programming and various Python libraries.
R Programming
R Programming is a programming language specifically designed for data analysis and statistical computing. It offers numerous statistical packages that are ideal for mode data analytics.
The key characteristic of R is its extensive array of open-source libraries dedicated to statistical analysis. These libraries provide sophisticated tools that can analyze datasets at an unprecedented level. Its unique feature lies in its ability to handle complex data sets and generate detailed graphical representations, which can be immensely beneficial in extracting the mode.
One of the advantages of using R is its vibrant community. Users can find a wealth of resources, from forums like reddit.com to comprehensive documentation. However, it does have a steeper learning curve for beginners, which can deter some potential users.
Python Libraries
Python is another powerhouse for data analytics, boasting libraries like pandas and NumPy which are widely used for processing data and calculating statistical metrics, including mode.
Pandas, with its ability to handle structured data, is particularly noted for its simplicity and efficiency. Users can easily manipulate data frames to find the mode and perform other statistical analyses. Additionally, Python libraries excel in their versatility, making it a popular choice among data scientists.
Nevertheless, using Python for mode analysis also has its drawbacks. While it is user-friendly, the performance may lag with exceedingly large datasets, and this could affect the accuracy of mode results.
Data Visualization Techniques
Visual representation of data is just as important as the analysis itself. Effective data visualization techniques help in communicating the results of mode analysis in a clear and impactful way.
Some common techniques include:
- Histograms: They provide a graphical representation of frequency distributions, helping to visualize where modes may lie in a dataset.
- Bar Charts: Useful for highlighting the frequency of occurrences of the mode, making it clear which values are dominant.
- Box Plots: These offer insights into the central tendency, spread, and potential outliers in a dataset.
By leveraging these tools and technologies, investors and analysts can draw insights that not only enhance their understanding of data but improve their decision-making strategies.
Case Studies: Mode Analytics in Practice
In the realm of mode data analytics, one cannot overlook the transformative impact of case studies. They serve as a bridge between theory and practice, shedding light on how the mode can guide decision-making in real-world scenarios. By examining specific examples, readers gain a clearer understanding of mode applications and the nuances that accompany them. Case studies provide invaluable insights that reinforce theoretical concepts, laying the groundwork for deeper comprehension in practical situations.
Real-World Applications
Case studies in mode analytics illustrate how businesses and professionals harness statistical measures to extract meaningful insights. Let's look at a few illustrative examples:
- Retail Sales Analysis: Imagine a retail chain analyzing its sales data to determine which products are the most popular among customers. By calculating the mode of sales figures over a specific period, the managers can pinpoint the best-selling items. This info allows them to manage inventory better, enhance marketing campaigns, and maximize profits.
- Customer Preferences: A restaurant may use mode data to discover the most preferred dish on its menu. By looking at order frequency data, the mode indicates the most ordered meals. This insight helps the restaurant fine-tune its offerings and promotions, ensuring they cater to their patrons' desires.
- Election Polling: In the political arena, mode analytics plays a critical role. By examining survey results from voters, pollsters can identify the most common preferences for candidates or policies, allowing campaign teams to adjust their strategies accordingly.
Each of these cases provides a glimpse into the diverse applications of mode data analytics, demonstrating its potential across various sectors. The beauty of using mode is its simplicity; it captures the most common occurrence, making it easily interpretable for stakeholders.
Lessons Learned from Data Analytics
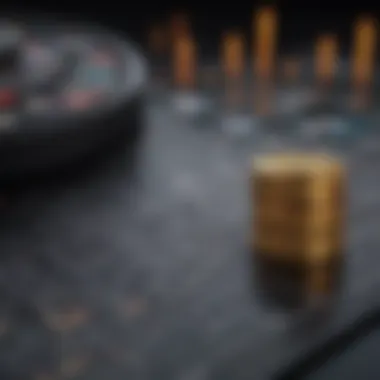
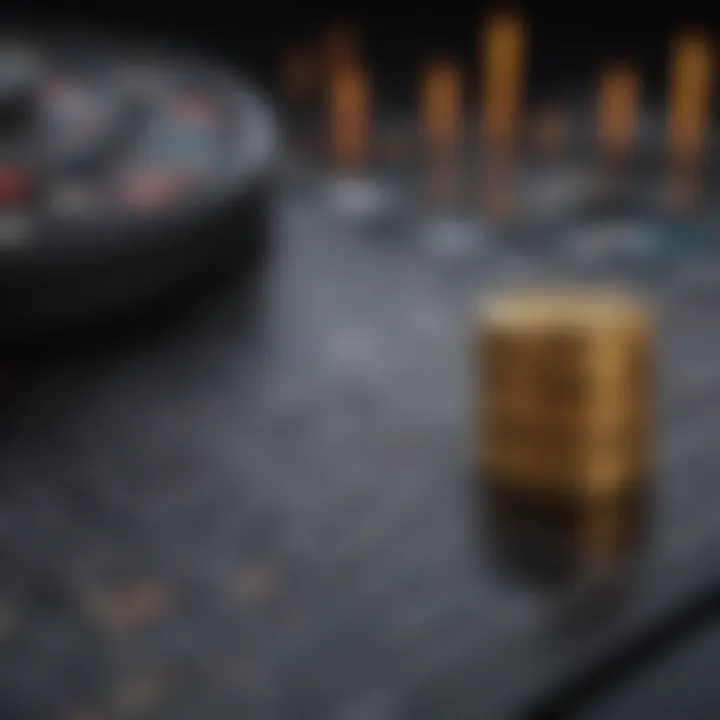
From engaging with mode analytics through case studies, several key takeaways emerge:
- Focus on the Significant: In the hustle of data mining, sometimes, the most obvious insights are overlooked. The mode, however, points out what’s most common, helping professionals focus on what truly matters.
- Diverse Data Is Essential: Often, organizations discover that they need to gather diverse data to make mode analytics valuable. A narrow dataset might lead to misleading conclusions. Thus, a broader perspective enriches analysis.
- Bias Awareness: When relying on mode, it's crucial to be mindful of how sample bias can skew results. For instance, if a poll mostly includes younger voters, the resulting mode may not accurately reflect the wider population's preferences.
"Data analytics isn't just about numbers; it's about understanding the stories behind those numbers."
- Adaptation: As industries evolve, so do the data they generate. Mode analytics must keep pace with these shifts to remain relevant. Organizations must be ready to adapt their strategies based on what the data shows.
Embracing these lessons not only solidifies the understanding of mode data analytics but also equips financial enthusiasts and individual investors with effective strategies for interpreting complex datasets. The clarity offered through case studies helps consolidate knowledge and approach data challenges with a fresh perspective.
Challenges in Mode Data Analytics
In the realm of data analytics, understanding the mode—a measure of central tendency—is crucial, yet it brings along its fair share of hurdles. These challenges can make the difference between extracting valuable insights and falling short. By diving into these complexities, investors and analysts alike can grasp the importance of rigorous data assessment. Addressing these challenges not only enhances the validity of the analysis but also builds a foundation for informed decision-making in finance and beyond.
Data Quality Issues
Data quality is the backbone of any analytic endeavor, particularly in mode analytics. Poor data quality can lead to misleading interpretations, which can have serious implications for financial strategies. Here are some key factors to consider:
- Incompleteness: Inadequate data collection methods can leave gaps that obscure the true mode. For instance, in financial market analysis, missing entries could misrepresent trends.
- Inconsistencies: Different sources may define or categorize data points in various formats. This inconsistency can result in skewed comparisons and ultimately misinformed decisions.
- Errors: Simple typographical errors in data entry, such as swapping digits or misclassifying variables, can heavily impact the calculation of the mode.
As an example, consider a retail store analyzing customer purchase behaviors. If data on specific products is recorded incorrectly, the mode of purchases derived might suggest that a certain product is favored when it actually isn’t, leading to misguided inventory decisions.
"Data is like garbage. You’d better know what you are going to do with it before you collect it." – Mark Twain
Cultural and Behavioral Influences
Beyond technical issues, cultural and behavioral factors play a significant role in how data is gathered and interpreted. These influences can create biases that affect mode analysis:
- Different Pricing Strategies: In various cultures, individuals may respond differently to pricing, leading to uneven purchasing patterns. This can distort the mode in financial analytics, as the true buying behavior might be obscured by these variations.
- Social Norms: In some cultures, collective behavior shapes how individuals respond to specific products or services, potentially inflating or deflating the perceived mode of these items. For instance, a product’s popularity in trendy areas can skew data far from the reality of broader customer preferences.
- Cognitive Biases: Analysts may inadvertently interpret data through a subjective lens. Confirmation bias or anchoring can lead to an overemphasis on certain data points, pushing the mode to represent a viewpoint that doesn’t accurately reflect overall trends.
Addressing these challenges requires not just careful data management but also an understanding of the intricate human behaviors that accompany data analytics. By recognizing the impact of these elements, professionals can refine their approach to mode analysis, improving outcomes and insights.
This comprehensive exploration of challenges in mode data analytics emphasizes the need for rigorous data practices and cultural awareness. Such a thorough understanding leads to better investments and enhances overall financial literacy, crucial for the savvy investor.
Future Trends in Mode Analytics
As the landscape of data analytics continues to evolve, understanding future trends in mode analytics becomes increasingly important. These trends have significant implications for decision-making in various fields—especially in finance, where timely and accurate data interpretation can shape investment strategies. The advancement of technology and data science methodologies creates opportunities for more nuanced insights from mode data, which can be a crucial element of financial analysis.-
Emerging Technologies
The emergence of new technologies in data collection and analysis has opened doors to previously untapped potential in mode analytics. Tools such as cloud computing facilitate the collection and storage of vast amounts of data, while advances in big data processing, like Apache Hadoop, allow analysts to handle large datasets efficiently. Consider the rise of no-code and low-code platforms that empower users without extensive technical skills to engage in mode analysis. These technologies usher in a more inclusive approach, broadening the base of professionals who can leverage mode analytics for insightful decision-making.
Moreover, real-time analytics technologies enable instant access to data trends, which significantly enhances the accuracy of mode calculations. By continuously updating metrics, investors can observe shifting patterns and make informed choices quicker than ever. For example, using Power BI or Tableau for mode analytics visualizations can provide stakeholders immediate insights and understanding of their datasets, laying bare the underlying trends in a clear, actionable manner.
Impact of Artificial Intelligence
Artificial Intelligence (AI) stands at the forefront of the next wave of advancements in mode analytics. By harnessing the power of machine learning algorithms, financial institutions can refine their ability to predict market trends based on historical mode data. These algorithms can scan through mountains of information, helping to uncover patterns that might not be visible to human analysts alone.
Investment firms are now implementing AI-driven predictive analysis, which assesses the mode along with other statistical measures, to develop more precise forecasts. One noteworthy application is in risk assessment—AI can learn from previous data to enhance the reliability of identified modes, potentially improving portfolio management strategies.
AI also plays a crucial role in automating data preparation processes. This reduces manual errors and facilitates more accurate computation of mode, allowing for deeper analyses that incorporate big data and alternative data sources. Thus, AI doesn't merely enhance existing capabilities; it transforms mode data analytics into a more adaptable and proactive tool for financial professionals who seek to stay ahead of the curve.
"The future of mode analytics will be intertwined with technology. Embracing emerging tools and AI capabilities is not just a trend; it’s a necessity for those who wish to thrive in the data-driven age."
Finale and Key Takeaways
In wrapping up our discourse on mode data analytics, it is essential to reflect on the various components that contribute to its overarching significance. This chapter is not just a summary, but it serves as a pivotal conduit within the article that connects theory to practice. Mode data analytics proves to be invaluable in being more than just a calculation; it embodies a tool that shapes decision-making and strategy in diverse fields.
Summarizing the Insights
Throughout the article, we unraveled how mode data, representing the most frequently occurring value within a data set, serves as a critical measure of central tendency. This statistical tool is often overshadowed by mean and median, yet its practical implications are profound, particularly when analyzing categorical data or identifying trends in consumer behavior.
"Understanding mode is like having a compass. It helps you navigate through the sea of data to find your true direction."
The insights gained underscore that mode analysis can unveil hidden patterns in data sets, be it in finance, retail, or social sciences. Some key points to note include:
- Mode’s simplicity allows for quick interpretations, making it extremely useful in initial data assessments.
- Its deployment in market analysis springs forth an understanding of popular trends, informing businesses about consumer preferences.
- Recognizing limitations alongside mode’s strengths further shapes comprehensive data analysis strategies.
Implications for Investors and Financial Professionals
For investors and financial professionals, mode data analytics provides a unique lens through which data can be scrutinized. Understanding the mode equips these stakeholders with the ability to make informed decisions based on existing trends, rather than relying solely on averages that may skew perspectives.
Consider the following benefits for financial decision-makers:
- Market Analysis: Identifying the mode can help in spotting prevailing market trends, guiding investment strategies effectively.
- Risk Assessment: By analyzing modes from different data sets, investors can gauge potential volatility and make calculated risks.
- Tailored Strategies: Mode data can inform personalized investment approaches, aligning strategies with prevailing consumer behavior data.
Every dollar invested involves a decision that could be modeled by the insights gleaned from these analyses. Thus, in a landscape dictated by numbers, understanding mode data analytics becomes akin to having a competitive edge. The implications for decision-making ripple far beyond individual investments, impacting broader market movements and economic trends.